PDF of this article (202 KB)

John Leathwick Malcolm Francis Paul Taylor
A new way of modelling trawl survey data is an important advance for mapping and protecting biodiversity.
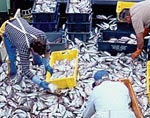


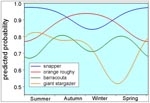
One of the great challenges of marine science is understanding what drives variation in the distribution of oceanic species and ecosystems. Although we can gain some insight by mapping species geographically, species distributions are generally determined primarily by environmental factors, such as depth, temperature and salinity. This raises the possibility of using statistical modelling tools to analyse the environental distributions of species, and recently we have explored this approach using catch data from research trawl surveys.
Selecting the variables
The first step in this project was to identify those environmental and biological factors likely to have functional links with species distributions, and to then find suitable data describing their spatial variation. For example, primary productivity is likely to provide a good measure of food availability, particularly for smaller fish; to capture spatial variation in this we used satellite imagery describing average chlorophyll concentrations at the sea surface. Similarly the frequent concentration of food resources along fronts where water masses meet was described using a satellite-based data layer that identifies areas with strong spatial gradients in sea-surface temperatures. We estimated temperature and salinity, which have a direct physiological effect on fish, at the depth of each trawl from the CSIRO Atlas of Regional Seas (CARS), a regional ocean climatology that describes 3-dimensional variation in water characteristics and includes coverage across the New Zealand region south to 50°S. Estimates of the strength of tidal currents were derived from the NIWA tidal model.
Applying the models
Statistical models were then used to analyse variation in the probability of capture for selected fish species as a function of the environmental factors described above. We selected 25 fish species with representatives from coastal through to deep offshore environments, including common and widespread species and some of restricted range. The Generalized Additive Models that we used accommodate presence–absence or count data and allow flexible model fitting. Once fitted, these models demonstrate how the probability of capture for a particular species varies in relation to, say, water temperature and salinity (see below). As expected, snapper show a high probability of capture in warmer, more saline waters of subtropical origin, which occur predominantly around coastal parts of northern New Zealand, whereas elephant fish are much more frequently caught in cooler, less saline waters, such as occur around coastal parts of the South Island. Hoki, although caught most frequently in cold waters, are also caught at moderate frequencies in waters with warmer temperatures.
Having established the environmental relationships for a species, we can export results from the statistical models to a geographic information system. There they are combined with maps of the various environmental factors to calculate and map probabilities of capture in geographic space. In the example shown above, predicted probabilities of capture for snapper, based on spatial variation in water temperature, salinity, chlorophyll a, sea-surface temperature gradients, sea-bed slope, and distance from the coast, coincide closely with the observed geographic distribution, indicating a good fit between the model and the underlying data.
Dealing with seasonal complexity
When we model the distributions of mobile organisms such as fish, the relationship between the catch rate for a species and the environment may vary depending on the time of year. This may reflect seasonal changes in behaviour within a particular environment, or perhaps seasonal migrations. We can accommodate this complexity by adding terms designed for circular data, which allows a curve to be fitted that describes the regular seasonal trend in capture rates. Examples of seasonal changes in catch rate modelled using this approach are shown in the graph.
Casting a wider net
The focus of this project has been to assess these analytical techniques for better understanding the distributions of marine fish. We would like to expand these analyses to improve the reliability of our environmental data, including information from a wider range of trawl surveys, and looking at a greater number of species. This will require improved information on spatial variation in water temperature and salinity, including for southern waters not covered by the CARS ocean climate model. We will also incorporate better estimates of ocean primary productivity, coastal re-suspension of sediments and freshwater inputs.
On the analytical side, we intend to explore the feasibility of modelling the amount of a species caught per unit effort, rather than just its probability of capture – initial trials with a few species show the potential of this approach, even with this limited set of data. We also plan to complete an analysis of variation in species richness in relation to environment, based on numbers of species caught in each research trawl.
Overall, we are impressed by the degree of predictability shown by most of the species we analysed, even when using a relatively restricted set of trawl data, and with environmental predictors of relatively low resolution. Our results show how carefully constructed statistical models can be used to gain insight into the relative importance of different environmental factors in driving biological distribution patterns in the oceans. So far, temperature at trawl depth emerges as the strongest predictor of capture probabilities for most species, followed by salinity and chlorophyll a concentration.
Continued development of this work has potential to contribute to the management of marine biodiversity by helping to identify areas with high species richness. Results will also support the ongoing development of environment-based classification frameworks of the seas around New Zealand. This will in turn provide a more robust basis for the objective definition and protection of representative marine ecosystems, and contribute to the sustainable harvest of resources.
Teachers’ resource for NCEA AS: Biology 2.9, 3.2; Science 2.2; Geography 2.4, 3.3. See other curriculum connections at www.niwa.co.nz/pubs/wa/resources
John Leathwick and Paul Taylor are based at NIWA in Hamilton; Malcolm Francis is at NIWA in Wellington.