University of Canterbury’s Professor Matthew Wilson, one of the Mā te haumaru ō te wai research team, gives us some insight on uncertainty.
Flood risk and other planning practitioners worldwide often use the outputs from flood modelling as part of their decision making. For instance, to determine flood hazard zones, design mitigation measures or assess the potential impacts of climate change on the flood hazard. However, these outputs contain uncertainty, which is not often quantified or characterised, yet can make the decision-making process more challenging and less reliable.
To account for uncertainty, planners may take a precautionary approach, such as adding a freeboard amount to required floor levels in flood zones or designing flood infrastructure such as stopbanks (levees) to a 1% annual exceedance probability (i.e., the 100-year average recurrence interval). However, this approach is questionable in an era of changing risk under climate change. For example, is the freeboard amount used sufficient to prevent serious damage from future floods? Will the area at flood risk increase? Will a current 100-year flood become a 50-year flood in future?
Some of these questions are aleatoric in nature: they will always be present and cannot be reduced. This includes issues such as the internal variability of the climate system, which implies that even if we had complete information about the future climate state, its chaotic nature means our flood risk assessments will still be uncertain. Other uncertainties are epistemic and are deterministic and subjective; the uncertainty contained in a flood risk assessment depends on how good (or bad!) the data are which are used within the analysis. Improving input data accuracy and model representations should, at least theoretically, reduce the inherent uncertainty in the predictions obtained and is something we always aim for.
Yet, even if we use the best possible data and model representations, uncertainty will still result from a complex combination of errors associated with source data, sampling and model representation. These uncertainties “cascade” through the risk assessment system (Figure 1a), reducing our confidence in any individual prediction and leading to variability in predicted depths and extents across multiple predictions which account for these errors. These uncertainties, here represented as variability in predicted depths and flows, further cascade through to the analysis of flood impacts (Figure 1b). Uncertainty in predicted depths and flows combine with errors from data such as those for buildings and infrastructure, and the statistical models used to quantify damage (e.g., via depth-damage curves). The end result is uncertainty in quantified damage for a flood scenario, creating issues for the decision-making processes such as determining whether to invest in improved mitigation measures.
This technical uncertainty is situated within social, economic and political contexts. While we wish to reduce uncertainty as much as possible (e.g., by using better data and models), it will always be present. If we do not develop a clear understanding of uncertainty, and communicate this appropriately, then this is likely to lead to doubts in both decision-makers and the wider public. For example, given uncertainty, a decision-maker may question how their decision would be received by others, or what might happen for different options, or whether their action would actually make a difference. The wider public is likely to question what this means for them: if it would flood again soon, if the next flood might be larger, if they should move, if they can get insurance and what happens if they can’t.
Although robust assessments of uncertainty would clearly be helpful, the need to run many thousands of simulations leads to a high computational demand and, consequently, they are not usually completed, especially at a national scale. In this research programme, one of our current PhD projects is developing a generalised uncertainty estimation method using machine learning which will enable rapid estimation of the reliability of flood risk estimates for sites, without the need to complete a full uncertainty analysis. A second PhD project is investigating the feasibility of using a hybrid hydrodynamic/ machine learning model to reduce the numerical modelling load and enable probabilistic modelling, to increase the number of scenarios which can be assessed, thereby enabling us to quantify uncertainties caused by our representation of storm rainfall. We will be soon be advertising a third uncertainty PhD project, which will focus on the implications of uncertainty in impact assessments on the planning process.
Further, we are engaging with regional and district councils regarding how we may best represent uncertainty within the maps and data outputs produced. If you are interested in engaging in this or any aspect of this important but challenging problem, please reach out to the project team.
Figure 1(a): Errors from multiple sources “cascades” through the analysis system, resulting in uncertainty in predicted depths and extents and the derived hazard assessment.
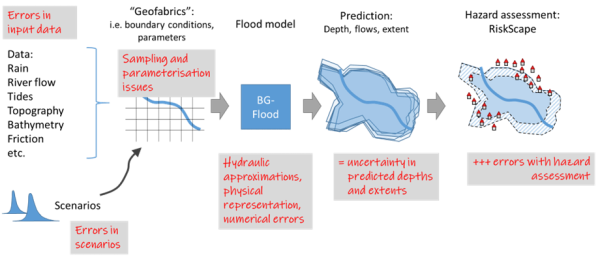
Figure 1(b): Analysis using the uncertain hazard assessment creates a further cascade, leading to uncertainty in the impact assessment and causing issues in decision making.
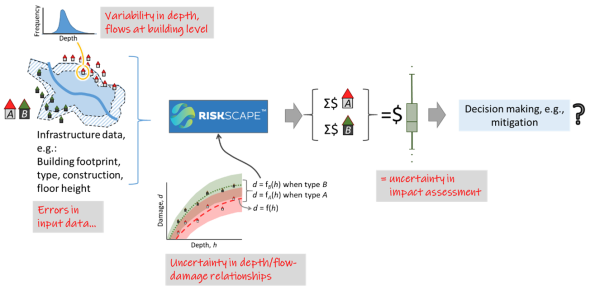